Uncharted Territory
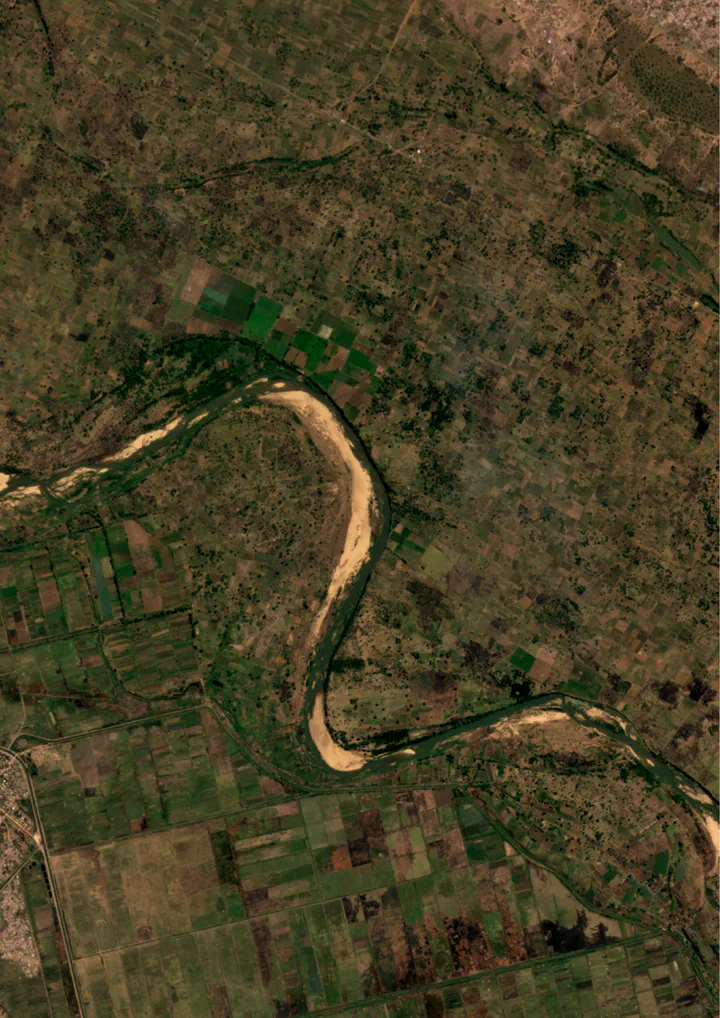
I wisely started with a map - J.R.R. Tolkien
This quote summarises how I start new projects, by thinking about the where, when, and how to show it. You would be surprised by how little maps are used in (project) proposals, yet how much they say (something about a thousand words, but times 10 because it’s spatial!).
What did I look at?
My biggest RS project so far – 4 years – is my PhD about mapping diverse and dispersed smallholder irrigation in sub-Saharan Africa through Remote Sensing.
Here are three options for you to read about it:
In recent years, there has been a renewed interest in irrigation in sub-Saharan Africa (SSA) due to the need for agricultural development and food security. Expanding irrigation is necessary to meet the region’s food requirements with the projected population growth. Smallholder farmers have long been driving irrigated agriculture in SSA for a long time through farmer-led irrigation development (FLID). Farmers have independently initiated, operated, and maintained irrigation infrastructure, often focusing on high-value cash crops to improve their income. However, FLID often goes unnoticed by official institutions due to its fragmented nature and the technical bias in defining irrigation. The small scale and heterogeneity of FLID make it challenging to accurately count and report official statistics. Moreover, the practices of smallholder farmers are sometimes considered inferior or irrelevant compared to “modern” irrigation technologies.
Similar challenges arise when mapping with remote sensing (RS) due to the complex and diverse nature of these systems. Several factors contribute to the difficulty in accurately measuring and classifying irrigated agriculture using satellite sensors. These factors include the similarity in spectral signatures between different land cover classes, mixed spectral signatures within the same land cover class, complex shapes and arrangements of fields, and subjective definitions of irrigation. Despite these challenges, RS offers several advantages for mapping irrigated agriculture. It provides wide spatial coverage, allows monitoring of temporal and spatial trends, and assists in prioritizing field visits. RS data can be consistently analysed over time and is easily accessible. Different classes of irrigated agriculture can be distinguished by considering factors such as the timing of image acquisition, variations in vegetation colour, and notable changes. This thesis aims to examine the production of remote sensing maps and their ability to depict irrigated agriculture. While remote sensing cannot directly measure farmer-led irrigation, it can capture the diverse and dispersed nature of small-scale irrigated agriculture, which requires interpretation through fieldwork and local expertise. The research identifies and addresses potential challenges in mapping irrigated agriculture in SSA using remote sensing data. The research uses four case studies in Mozambique, specifically Chokwe, Xai-Xai, Manica, and Catandica, chosen for their diverse agroecological characteristics and the presence of both small-scale and large-scale irrigated agriculture. In Chapter 2, I look at common RS classification steps that all mapping studies go through. I developed a framework to explicitly address and assess modelling choices, covering seven steps that all classification studies typically go through. The framework aims to evaluate the reproducibility of results across different studies. The primary results highlight two key findings. Firstly, the study demonstrates and systematizes the impact of different choices on the classification process. Secondly, it reveals a concerning culture of insufficient reporting on eight crucial choices. The lack of reporting in these eight domains suggests a potential lack of awareness among map makers regarding the significance of their methodological choices in accurately defining the extent of irrigated agriculture and reproducibility. Consequently, the produced maps likely underreport the full extent of irrigated agriculture, especially that of smallholder farmers.
In Chapter 3, I examined how different algorithms and composite lengths affect the accuracy of predicting irrigated agriculture in Mozambique. Composites are commonly used to generate cloud-free and spatially consistent images from satellite time series by aggregating summary measures from the time series, such as the mean pixel value. Creating composites on a monthly, seasonal, or annual basis can effectively capture vegetation phenology. Specifically, I evaluated how four classifiers (the random forest (RF), support vector machine (SVM), artificial neural networks (ANN), and k-nearest neighbours (k-NN)) and four composite lengths (1 × 12-monthly, 2 × 6-monthly, 4 × 3-monthly, and 6 × 2-monthly) classified irrigated agriculture. I present the results using “agreement maps” that illustrate the consensus among the models regarding the classification of an area as irrigated agriculture or non-irrigated. These maps highlight the presence of core areas of irrigated agriculture, known as hotspots, which exhibit a high level of certainty. Surrounding these hotspots is an uncertainty zone where the models exhibit less agreement. These maps can combine the strengths of multiple models and reduce the possibility of false positives (areas incorrectly classified as irrigated agriculture). I found that artificial ANN, SVM, and RF all performed effectively in classifying irrigated areas. However, there was no single “best” algorithm. For complex and heterogeneous landscapes, shorter composites are found to be more suitable. Conversely, longer composites are sufficient for more uniform landscapes. Promising options, such as 6-month and 3-month composites, offer advantages in reduced computation time and data size while still achieving high classification accuracy. My analysis demonstrates that combining models with different composite lengths and algorithms into agreement maps improves the accuracy of identifying irrigated agriculture. Chapter 4 centres on the impact of training sample size and composition on the accuracy of RS classification for mapping smallholder irrigated agriculture in SSA. In particular, I investigate the optimal number of samples, their quality, and the class imbalance issue. Collecting extensive and high-quality training samples presents difficulties due to limitations in time, access and interpretability. As a result, class imbalance, where certain classes are more abundant in the training data, can lead to challenges in accurately classifying minority classes. The available sample size can affect the choice of algorithm, as some algorithms require a larger dataset than others. These challenges are particularly relevant in the context of smallholder irrigated agriculture, as it is often underrepresented in datasets and policies. In addition to the dataset’s size, training data biases can affect classification outcomes. These biases can arise from limited local knowledge, mislabelling, and the human aspect of interpretation. The various explored scenarios of Chapter 4 show that larger sample sizes generally improve user and producer accuracies; these are class-specific accuracies that can be used to show if that class is over- or underestimated. However, there is a point of diminishing returns where further increases in sample size only marginally increase accuracy and require more resources. The study also reveals that models trained on Gaza perform better overall, indicating a more generalized model compared to the overfitting observed in Manica; in other words, the Gaza model was better able to predict all classes without much preference towards single classes. In contrast, the Manica model favoured irrigated agriculture more than other classes. Other scenarios highlight the importance of collecting representative field data and using suitable algorithms, such as RF and SVM, which are less sensitive to specific dataset characteristics compared to the ANN. Chapter 5 investigates whether transferring models between regions can improve model performance and save resources compared to collecting new data. I hypothesize that targeted data collection is necessary in the new area since the relationships between spectral responses and land covers learned in one area may not apply due to variations in weather conditions, landscapes, and farming practices. Instead of random data collection, I focused on identifying areas with high prediction errors to guide targeted data collection efforts. Various models were trained on data from different scenarios to investigate the potential transferability of machine learning models for predicting irrigated agriculture. The study found that simple transfers of models were not effective in correctly classifying new areas due to insufficient training data. However, incorporating more diverse data from multiple regions improved the classification performance. Unsurprisingly, the best results were achieved when using only data from the target area, excluding data from other areas. To conclude, the field of remote sensing-based land use/land cover classifications has been democratised due to various factors, including the availability of open-source software like QGIS and R, open data policies by organizations such as Landsat, MODIS, and Sentinel, as well as the emergence of cloud computing platforms like Google Earth Engine and Digital Earth Africa. Additionally, online tutorials and platforms such as GitHub have made RS techniques more accessible and widely adopted. This accessibility has empowered individuals and smaller groups who previously lacked the resources to engage in mapping activities. However, the diversity of methods and (research) objectives used in creating these maps poses a challenge: it is not always straightforward what methods to use or not, what to report on, and extrapolating the results to other cases. The results of this research have implications for documenting and reporting of methods and choices, presenting irrigated agriculture through maps, and showing how easy it is to manipulate those maps with slight tweaks to models.Long story short :)
Short story long :)
My coding journey
Although I had some courses in R during my studies, I learned most after it. I had to deal with finding the data in the first place, organising field data collection (where do the enumerators go specifically), make sure it was reproducible, scalable, etc. etc. None of these topics were really discussed at university. So the first two years was a lot of trial and error, but it gave me a lot of room to play around, experiment with different packages, and finally to build it in such a way that I could turn on the models, and come back after a few days with all the maps ready for me to look at.
Now we all know about AI to make all of this code, but ‘back in my day’ (pre-ChatGPT… ) I had to search the internet, stack exchange, and hope that authors published their code (which is not often). Lots of copying and adjusting code, figuring out how to speed up code, etc. Good thing R is open course, and many people who use it give back.
And that is also the reason for this website. I want to experiment with sharing code and insights in mapping irrigation, in the hope that someday somebody will find something useful here. And as this website is made in R, I gave myself a new toy to play around with :D.
Proudest part of code
I think I’m most proud of this piece of code. This R function model_function_ffs
trains and evaluates machine learning models for spatial data analysis, specifically focusing on predicting classes (code_level2
) and uses various algorithms such as k-nearest neighbors (knn), neural networks (nnet), support vector machines with radial kernel (svmRadial), and random forests (rf). Full code here.
Click to see the function `model_functions_ffs`
model_function_ffs <- function(composite, composite_lengths, algorithm){
set.seed(100)
polys_split <- initial_split(data = TD_df, prop = .8, strata = code_level2) #prop defines the amount of split #I chose not to split based on collection method, the data was just not good enough to do that
TD_df_training <- training(polys_split)
head(TD_df_training)
# model training
predictors <- names(composite)
response <- "code_level2"
trainDat <- training(polys_split)
indices <- CreateSpacetimeFolds(trainDat,
spacevar = "PolygonID",
k=3,
class="code_level2")
trainDat <- trainDat %>% select(-PolygonID)
trainDat <- mutate(trainDat, code_level2 = as.factor(code_level2))
no_cores <- detectCores() - 2
cl <- makeCluster(no_cores)
registerDoParallel(cl)
set.seed = 100
if(algorithm == "knn"){ # knn does not work in parallel mode
ctrl <- trainControl(method="cv",
index = indices$index,
savePredictions = TRUE,
allowParallel= F,
number = 5,
verboseIter = TRUE)
}else{
ctrl <- trainControl(method="cv",
index = indices$index,
savePredictions = TRUE,
allowParallel= TRUE,
number = 5,
verboseIter = TRUE)}
# num of models = 2x(n-1)^2/2
n <- nlayers(composite)
print(2*(n-1)^2/2)
if(algorithm == "knn"){
print("knn model")
model_ffs <- ffs( trainDat[,predictors],
trainDat[,response],
method=algorithm,
metric="Accuracy",
trControl=ctrl,
tuneLength = 5,
preProcess = c("center", "scale")
)
}else if(algorithm == "nnet"){
print("nnet model")
model_ffs <- ffs( trainDat[,predictors],
trainDat[,response],
method=algorithm,
metric="Accuracy",
trControl=ctrl,
tuneLength = 10,
preProcess = c("center", "scale")
)
}else if(algorithm == "svmRadial"){ #svmRadial prediction does not work in ffs mode, so I select the variables using ffs, and do a second training thorugh 'train'
print("svm model")
model_ffs_varselect <- ffs( trainDat[,predictors],
trainDat[,response],
method=algorithm,
metric="Accuracy",
trControl=ctrl,
importance=TRUE,
withinSE = TRUE,
tuneLength = 5,
na.rm = TRUE ,
preProcess = c("center", "scale"))
SVM_radial_vars <- c(model_ffs_varselect$selectedvars ,'code_level2') #here I select only the variables from the ffs output
trainDat2 <- trainDat %>% select(all_of(SVM_radial_vars))
model_ffs <- train(
code_level2 ~ .,
data = trainDat2,
method=algorithm,
metric="Accuracy",
trControl=ctrl,
importance=TRUE,
withinSE = TRUE,
tuneLength = 5,
na.rm = TRUE ,
preProcess = c("center", "scale"))
} else if(algorithm == "rf"){ #no need to centre or scale RF input data, see discussion: https://stackoverflow.com/questions/8961586/do-i-need-to-normalize-or-scale-data-for-randomforest-r-package
print( "rf model")
model_ffs <- ffs( trainDat[,predictors],
trainDat[,response],
method=algorithm,
metric="Accuracy",
trControl=ctrl,
importance=TRUE,
withinSE = TRUE,
tuneLength = 5,
na.rm = TRUE
)}
model_ffs <- readRDS(here("output", "Maps", "round_two","Models", paste0(algorithm,"_train_", location, "_", composite_lengths, ".rds")))
saveRDS(model_ffs, here("output", "Maps", "round_two","Models", paste0(algorithm,"_ffs_", location, "_", composite_lengths, ".rds")))
prediction_ffs_rf <- predict(object = raster_ready, model = model_ffs, progress = "text", cores = no_cores)
writeRaster(prediction_ffs_rf, here("output", "Maps", "round_two", "Maps", paste0(algorithm, "_train_", location, "_", composite_lengths, ".tif")),overwrite=TRUE)
}
This allowed me to classify any area simply by running model_function_ffs(raster_ready_12m, "12m", "rf")
for example. Although it may seem like a small function (with sub-functions), when I figured this out and was able to let me laptop run over all study areas and combinations of data and algorithms, I was happ: stuff is happening whilst I can sit outside and enjoy the sun or play lacrosse :).